Article • 1 min read
What is AI transparency? A comprehensive guide
AI transparency builds trust, ensures fairness, and complies with regulations. Dive into the benefits, challenges, and strategies to achieve transparency in AI.
By Hannah Wren, Staff writer
Last updated January 18, 2024
What is AI transparency?
AI transparency means understanding how artificial intelligence systems make decisions, why they produce specific results, and what data they’re using. Simply put, AI transparency is like providing a window into the inner workings of AI, helping people understand and trust how these systems work.
We use artificial intelligence (AI) more than we think—some of us speak with Siri or Alexa every day. As we continue to learn more about the impact of AI, businesses must keep transparency in AI top of mind, especially when it comes to the customer experience (CX).
Taking facts and figures from our Zendesk Customer Experience Trends Report 2024, our beginner’s guide to transparent AI includes the significance of AI transparency and its requirements, regulations, benefits, challenges, best practices, and more.
More in this guide:
- Why is AI transparency important?
- AI transparency requirements
- Levels of AI transparency
- Regulations and standards of transparency in AI
- The benefits of AI transparency
- Challenges of transparency in AI (and ways to address them)
- AI transparency best practices
- Examples of companies practicing transparent AI
- Frequently asked questions
- What’s next for AI transparency?
Why is AI transparency important?
In the most simplified terms, transparency in AI is important because it provides a clear explanation for why things happen with AI. It helps us understand the reasons behind AI’s decisions and actions so we can ensure they’re fair and reliable. According to our CX Trends Report, 65 percent of CX leaders see AI as a strategic necessity, making AI transparency a crucial element to consider.
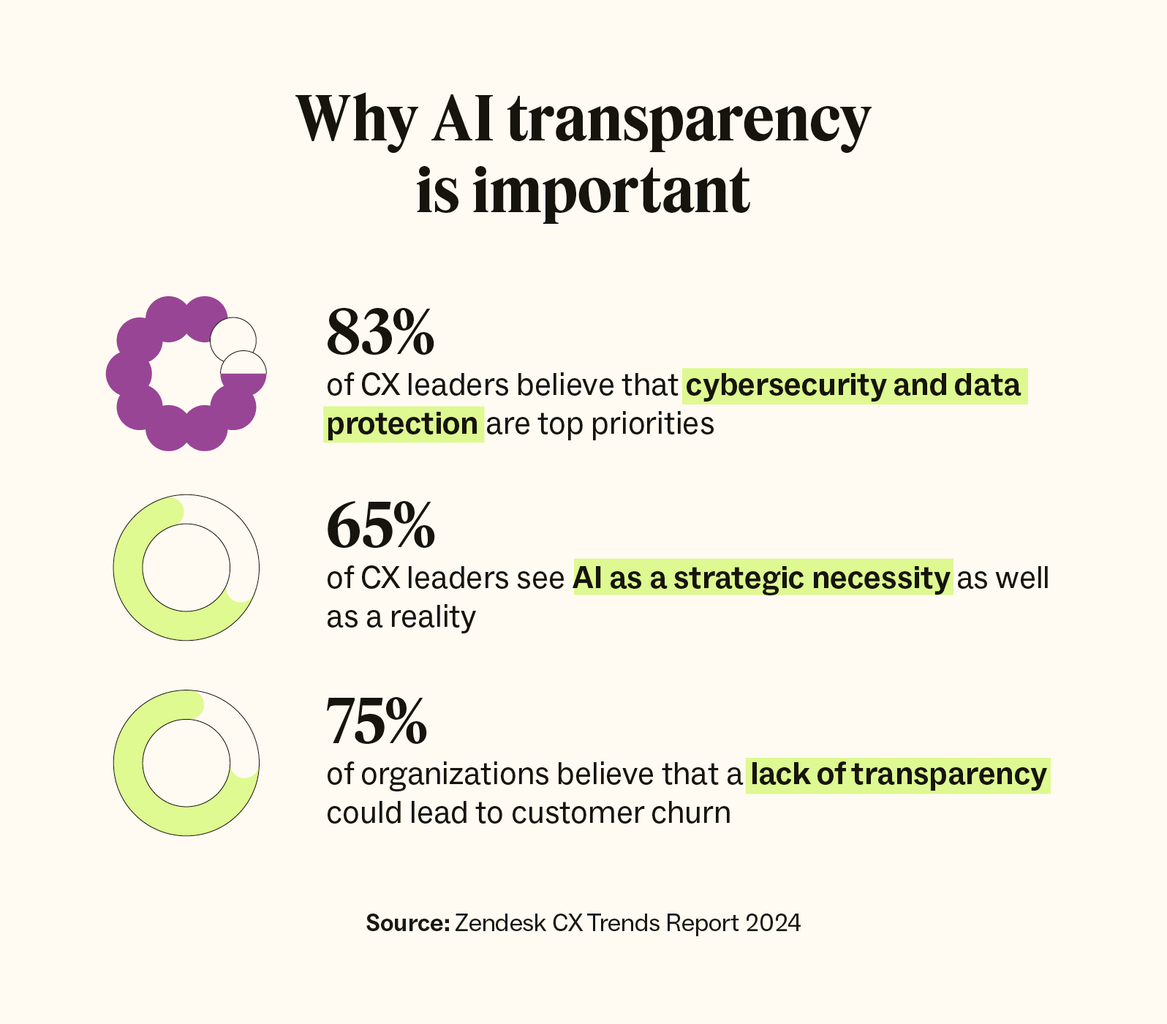
Being transparent about the data that drives AI models and their decisions will be a defining element in building and maintaining trust with customers.Zendesk CX Trends Report 2024
AI transparency involves understanding its ethical, legal, and societal implications and how transparency fosters trust with users and stakeholders. According to our CX Trends Report, 75 percent of businesses believe that a lack of transparency could lead to increased customer churn in the future. Because AI as a service (AIaaS) providers make AI technology more accessible to businesses, ensuring AI transparency is more important than ever.
The ethical implications of AI means making sure AI behaves fairly and responsibly. Biases in AI models can unintentionally discriminate against certain demographics. For example, using AI in the workplace can help with the hiring process, but it may inadvertently favor certain groups over others based on irrelevant factors like gender or race. Transparent AI helps reduce biases to sustain fair results in business use cases.
The legal Implications of AI involve ensuring that AI systems follow the rules and laws set by governments. For instance, if an AI-powered software collects personal information without proper consent, it can violate privacy laws. Creating laws that emphasize transparency in AI can ensure compliance with legal requirements.
The societal implications of AI entail understanding how AI affects the daily lives of individuals and society as a whole. For example, using AI in healthcare can help doctors make accurate diagnoses faster or suggest personalized treatments. However, it can raise questions about equitable access based on the technology’s affordability.
AI transparency requirements
There are three key requirements for transparent AI: explainability, interpretability, and accountability. Let’s look at what these requirements are and how they pertain to training data, algorithms, and decision-making in AI.
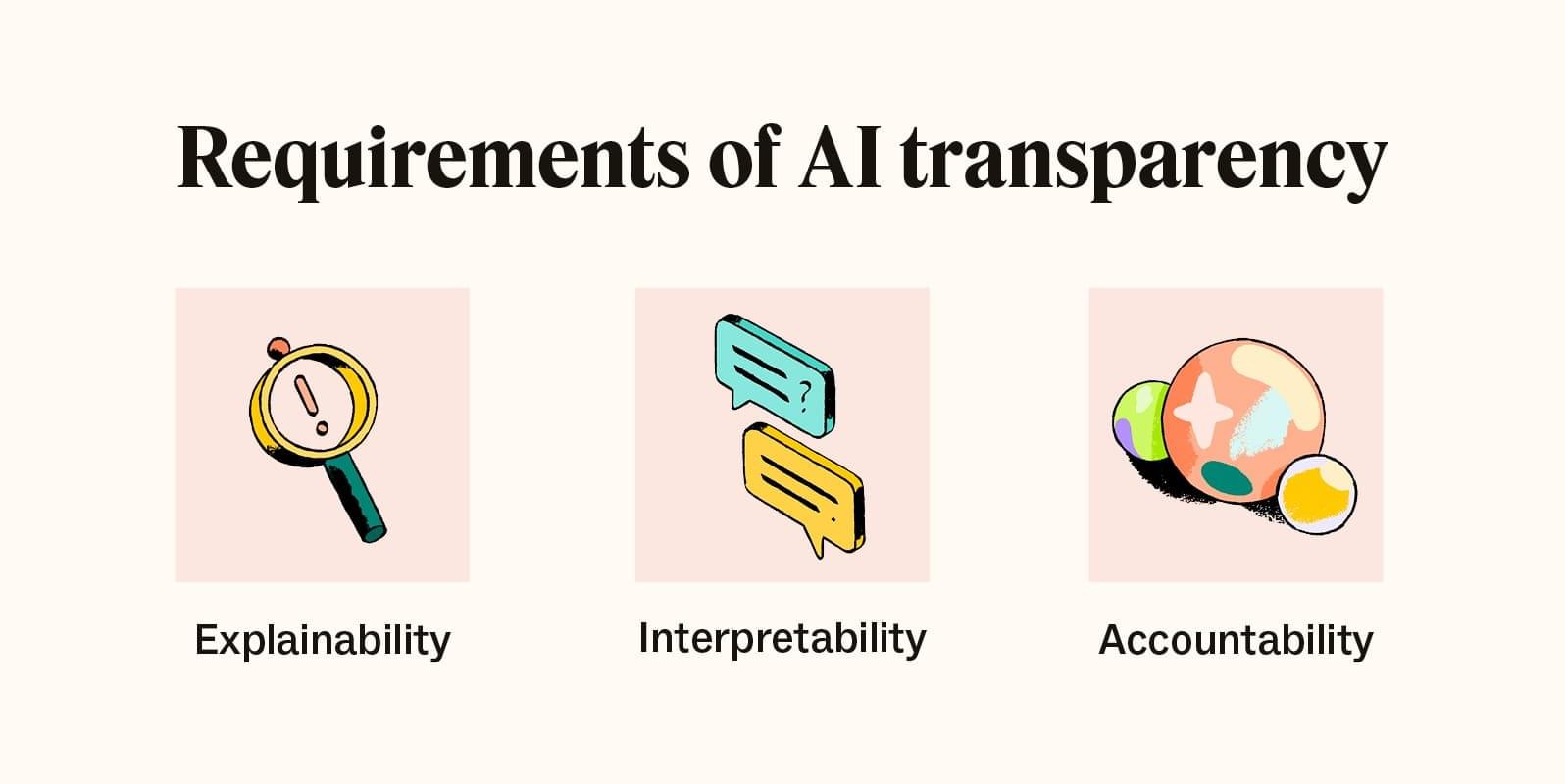
Explainability
Explainable AI (XAI) refers to the ability of an AI system to provide easy-to-understand explanations for its decisions and actions. For example, if a customer asks a chatbot for product recommendations, an explainable AI system could provide details such as:
“We think you’d like this product based on your purchase history and preferences.”
“We’re recommending this product based on your positive reviews for similar items.”
Offering clear explanations gives the customer an understanding of the AI’s decision-making process. This builds customer trust because consumers understand what’s behind the AI’s responses. This concept can also be referred to as responsible AI, trustworthy AI, or glass box systems.
On the flip side, there are black box systems. These AI models are complex and provide results without clearly explaining how they achieved them. This lack of transparency makes it difficult or impossible for users to understand the AI’s decision-making processes, leading to a lack of trust in the information provided.
Interpretability
Interpretability in AI focuses on human understanding of how an AI model operates and behaves. While XAI focuses on providing clear explanations about the results, interpretability focuses on internal processes (like the relationships between inputs and outputs) to understand the system’s predictions or decisions.
Let’s use the same scenario from above where a customer asks a chatbot for product suggestions. An interpretable AI system could explain that it uses a decision tree model to decide on a recommendation.
Accountability
Accountability in AI means ensuring AI systems are held responsible for their actions and decisions. With machine learning (ML), AI should learn from its mistakes and improve over time, while businesses should take suitable corrective actions to prevent similar errors in the future.
Say an AI chatbot mistakenly recommends an item that’s out of stock. The customer attempts to purchase the product because they believe it’s available, but they are later informed that the item is temporarily out of stock, leading to frustration. The company apologizes and implements human oversight to review and validate critical product-related information before bots can communicate it to customers.
This example of accountability in AI for customer service shows how the company took responsibility for the error, outlined steps to correct it, and implemented preventative measures. Businesses should also perform regular audits of AI systems to identify and eliminate biases, ensure fair and nondiscriminatory outcomes, and foster transparency in AI.
Levels of AI transparency
There are three levels of AI transparency, starting from within the AI system, then moving to the user, and finishing with a global impact. The levels are as follows:
Algorithmic transparency
Interaction transparency
Social transparency
Algorithmic transparency focuses on explaining the logic, processes, and algorithms used by AI systems. It provides insights into the types of AI algorithms, like machine learning models, decision trees (flowchart-like models), neural networks (computational models), and more. It also details how systems process data, how they reach decisions, and any factors that influence those decisions. This level of transparency makes the internal workings of AI models more understandable to users and stakeholders.
Interaction transparency deals with the communication and interactions between users and AI systems. It involves making exchanges more transparent and understandable. Businesses can achieve this by creating interfaces that communicate how the AI system operates and what users can expect from their interactions.
Social transparency extends beyond the technical aspects and focuses on the broader impact of AI systems on society as a whole. This level of transparency addresses the ethical and societal implications of AI deployment, including potential biases, fairness, and privacy concerns.
Regulations and standards of transparency in AI
Because artificial intelligence is a newer technology, the regulations and standards of transparency in AI have been rapidly evolving to address ethical, legal, and societal concerns. Here are a few key regulations and standards to help govern artificial intelligence:
- General Data Protection Regulation (GDPR): established by the European Union (EU) and includes provisions surrounding data protection, privacy, consent, and transparency
- Organisation for Economic Co-operation and Development (OECD) AI Principles: a set of value-based principles that promotes the trustworthy, transparent, explainable, accountable, and secure use of AI
- U.S. Government Accountability Office (GAO) AI accountability framework: a framework that outlines responsibilities and liabilities in AI systems, ensuring accountability and transparency for AI-generated results
- EU Artificial Intelligence Act: an act proposed by the European Commission that aims to regulate the development of AI systems in the EU
These regulations can standardize the use and development of AI, locally and globally. AI systems can be consistently more clear and trustworthy by emphasizing transparency, ethical considerations, and accountability.
The benefits of AI transparency
Transparent AI offers many benefits for businesses across ethical, operational, and societal realms. Here are a few advantages of transparency in AI:
- Builds trust with users, customers, and stakeholders: Users, customers, and stakeholders are more likely to engage with AI technologies or businesses that utilize an AI help desk when they understand how these systems function and trust that they operate fairly and ethically.
- Promotes accountability and responsible use of AI: Clear documentation and explanations of AI processes make the responsible use of AI easier and hold businesses accountable in case of errors or biases.
- Detects and mitigates data biases and discrimination: Visibility into the data sources and algorithms allows developers and data scientists to identify biases and discriminatory patterns. This allows businesses to take proactive steps to eliminate biases and ensure fair, equitable outcomes.
- Improves AI performance: Developers who clearly understand how models operate can fine-tune algorithms and processes more effectively. Feedback collected from users and insights from performance data allow for continuous improvements to enhance the accuracy and efficiency of AI systems over time, especially with AI for the employee experience.
- Addresses ethical issues and concerns: Transparency in AI enables stakeholders to evaluate the ethical implications of AI-powered decisions and actions and ensure that AI systems operate within ethical guidelines.
Embracing transparency in AI not only enhances the reliability of AI systems but also contributes to responsible and ethical usage.
AI trends for CX in 2024
Implementing AI-powered tools into customer service software enhances the customer experience. But we’ve only just begun to scratch the surface. Check out how you can unlock the power of AI by downloading the Zendesk Customer Experience Trends Report 2024.
Challenges of transparency in AI (and ways to address them)
Along with the many benefits of AI transparency come a few challenges. These challenges, however, can be managed and minimized effectively.
Keeping data secure
Ensuring customer data privacy while maintaining transparency can be a balancing act. Transparency may require sharing details about the data used in AI software, raising concerns about data privacy. According to our CX Trends Report, 83 percent of CX leaders say data protection and cybersecurity are top priorities in their customer service strategies.
How to handle this challenge:
Appoint at least one person on the team whose primary responsibility is data protection. Brandon Tidd, the lead Zendesk architect at 729 Solutions, says that “CX leaders must critically think about their entry and exit points and actively workshop scenarios wherein a bad actor may attempt to compromise your systems.”
Explaining complex AI models
Some AI models, especially those utilizing deep learning or neural networks, can be challenging to explain in simple terms. This makes it difficult for users to grasp complex AI models’ decision-making and intelligent automation processes.
How to handle this challenge:
Develop visuals or simplified diagrams to illustrate how complex AI models function. Choose an AI-powered software with a user-friendly interface that provides easy-to-follow explanations without the technical stuff.
Maintaining transparency with evolving AI models
As AI models change and adapt over time, maintaining transparency becomes increasingly more difficult. Making updates or modifications to AI systems or retraining them on new datasets can alter their decision-making processes, which can make it challenging to maintain transparency consistently.
How to handle this challenge:
Establish a comprehensive documentation process that tracks the changes made to an AI ecosystem, like its algorithms and data. Provide regular and updated transparency reports that note these changes in the AI system so stakeholders are informed about these updates and any implications.
AI transparency best practices
Incorporating AI transparency best practices helps foster accountability and trust between AI developers, businesses, and users. Clear and open communication about data practices, bias prevention measures, and the data used (and not used) in AI models can help users feel more confident using AI technology. Here are some best practices for ensuring transparent AI.
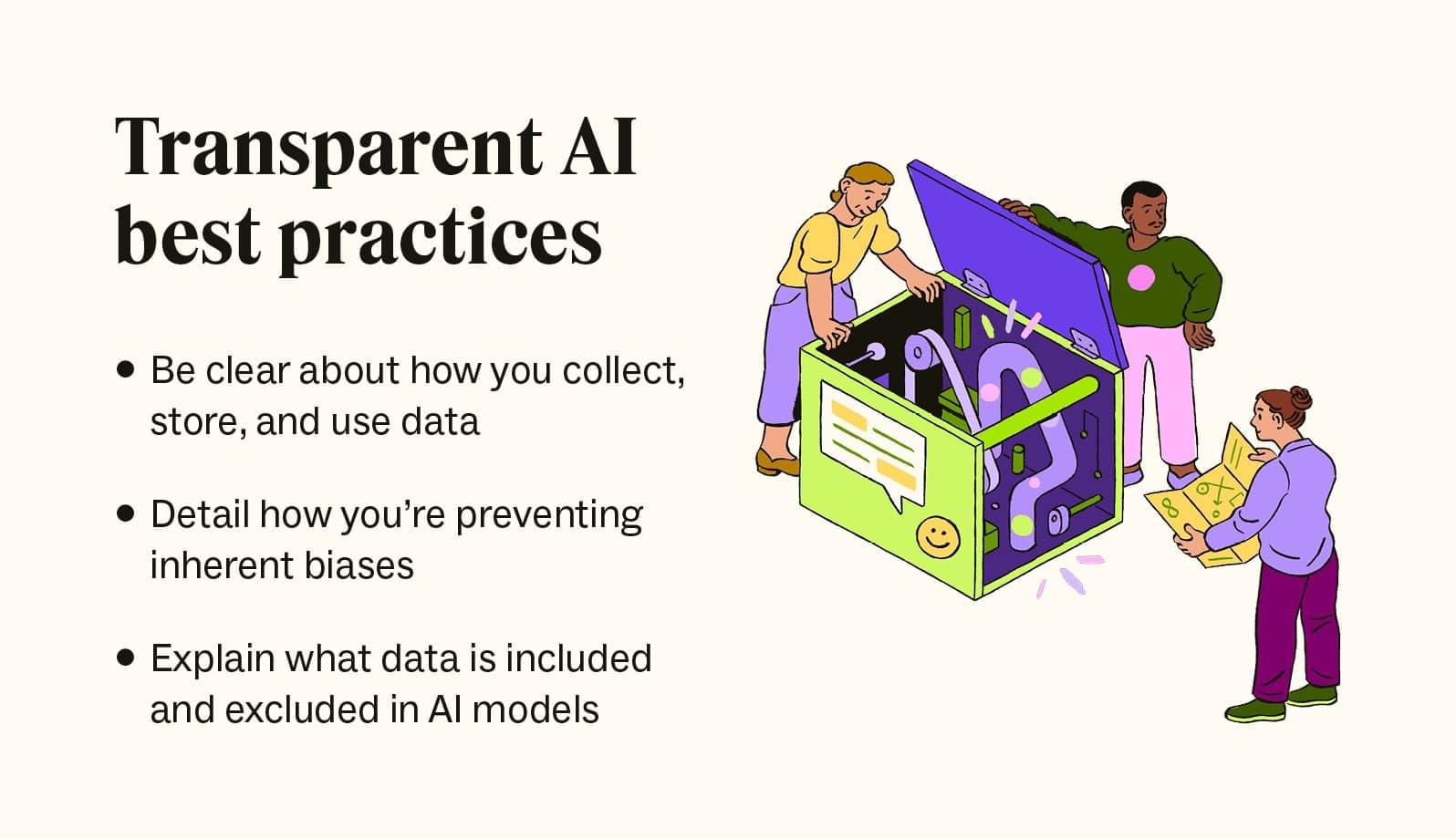
Be clear with customers about how their data is collected, stored, and used
Provide transparent and understandable explanations to customers about the collection, storage, and utilization of their data by AI systems. Clearly outline privacy policies detailing the type of data collected, the purpose of collection, storage methods, and data usage in AI systems. Protecting customer privacy starts with obtaining explicit consent from users before collecting or using their data for AI purposes.
Detail how you’re preventing inherent biases
Conduct regular assessments to identify and eliminate biases within your AI software. Communicate the methods used to prevent and address biases in AI models so users understand the steps being taken to enhance fairness and prevent discrimination. Maintain records of bias detection, evaluation, and processes to show a commitment to customer transparency and bias prevention.
Explain what data is included and excluded in AI models
Clearly define and communicate the types of data included and excluded from AI models. Provide reasoning behind the selection of data used in AI training to help users understand the model’s limitations and capabilities. Avoid including sensitive or discriminatory data that could result in biases or infringe on privacy rights.
Examples of companies practicing transparent AI
Here are a few examples of companies putting transparent AI initiatives into action. Their efforts to maintain AI transparency and promote responsible AI practices continue to help them build trust with their customers.
Zendesk
At Zendesk, we create customer experience software that enables users to enhance their customer support with AI and machine learning tools, like generative AI and AI chatbots. Zendesk AI emphasizes explainability by providing insights into how its AI-powered tools work and how AI decisions are made.
Zendesk also offers educational resources and documentation to help users understand AI’s integration into customer service software, the ethics of AI in CX, and its impact on customer interactions.
Lush
Cosmetic retailer Lush is vocal about ethical AI usage in its business operations. The company is transparent about not using social scoring systems or technologies that could infringe on customer privacy or autonomy. Lush engages in public discussions and shares its stance on ethical AI practices through its communications and social media channels.
OpenAI
OpenAI, an AI research laboratory popular for its generative AI applications ChatGPT and Dall-E, regularly publishes research papers and findings that provide insights into its AI developments and breakthroughs.
OpenAI is transparent about its goals, ethical guidelines, and the potential societal impact of AI through comprehensive documentation. The company encourages collaboration and engagement with the wider AI community, fostering transparency and sharing knowledge about AI development.
65% percent of CX leaders see AI not as a fad but as a strategic necessity and reality.Zendesk CX Trends Report 2024
Frequently asked questions
What’s next for AI transparency?
As artificial intelligence continues to evolve and advance, so will transparency in AI. Though it may be difficult to predict exactly what the future of AI transparency will look like, several trends and expectations might shape the landscape.
These expectations include developing better tools to help explain complex AI models so users can understand the AI decision-making process, which will help to increase trust and usability.
There will also be more emphasis on AI regulations and ethical considerations. This will make it easier for businesses to implement standard practices for AI transparency, addressing biases, fairness, and privacy concerns for more responsible AI systems.